Generative Pre-trained Transformer or GPT AI Models Explained: How Does GPT and ChatGPT work?
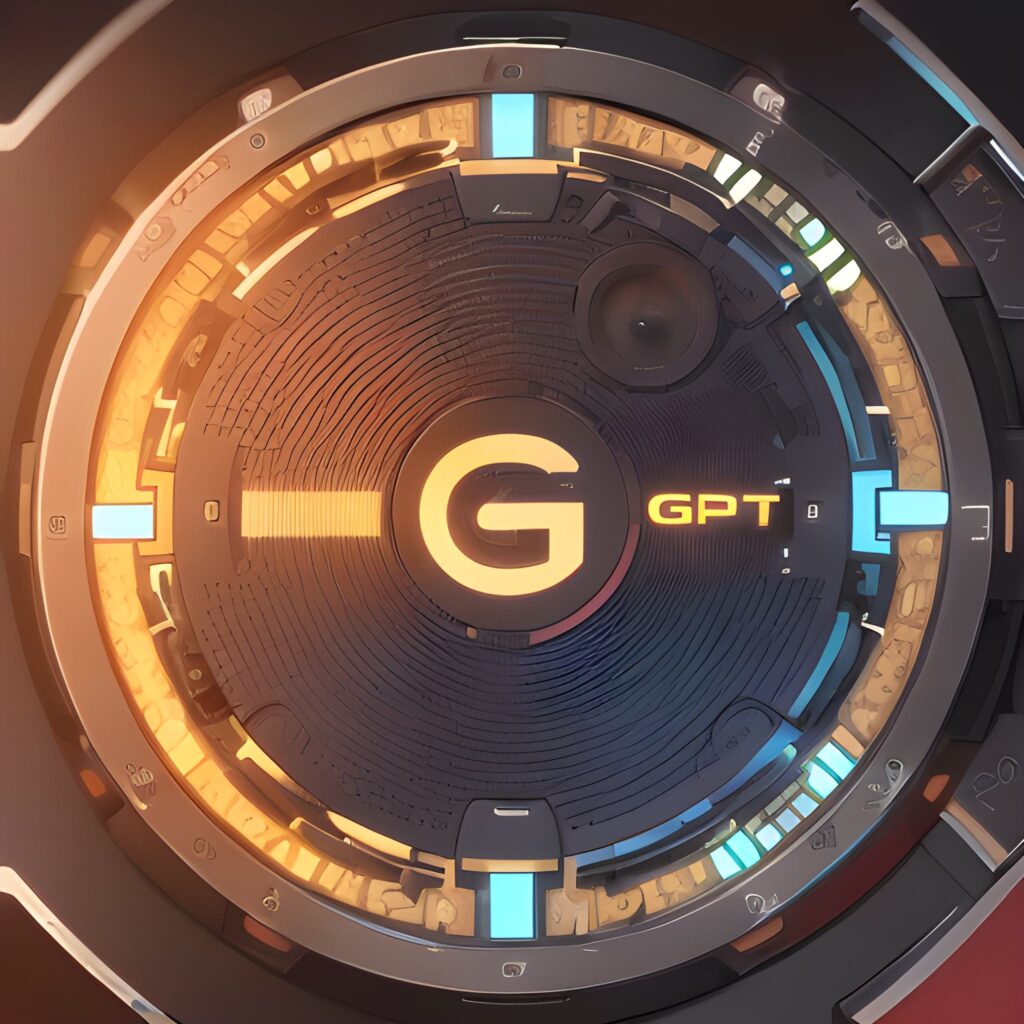
How Does GPT and ChatGPT work?, deepleaps.com, 4k, 8k, ultra highres, raw photo in hdr, sharp focus, intricate texture
You might have heard of and by today used amazing AI programs like OpenAI’s ChatGPT, GPT-3, GPT-4, Microsoft’s Bing Chat, Facebook’s LLaMa, or Google’s Bard. They can talk like humans and do lots of different tasks, such as writing essays or creating websites. But how do these AI programs work? Let’s take a look at how they can have conversations with us.
ChatGPT and similar AI programs can talk to us because they use advanced technology and a lot of training. They’re built using something called “transformer architecture,” which helps them understand and process lots of text data. This helps the AI learn about language and how words and phrases are connected.
To teach the AI, it’s given a huge amount of text from things like books, articles, and websites. This way, the AI learns grammar, sentence structure, and lots of information about many different topics. With all this knowledge, the AI can give accurate and sensible answers during conversations.
After the AI is trained, it can read the text you give it and guess what words should come next. It does this by thinking about the words it has seen before and the patterns it has learned. The AI then picks the best answer based on what it thinks is most likely, making it seem like it’s having a human-like conversation.
These AI programs are impressive because they can talk like humans and do many different tasks. As we keep learning more about ChatGPT and other AI models, they will keep getting better, and we can expect even more exciting uses for AI in our daily lives.
A Simple Look at How Language AI Learns: Using Probabilities
A basic way to teach AI about language is using a method called a Markov chain. It’s a simple idea where the AI learns from a text and looks at the chances of words coming after one another. Let’s use this example sentence:
The cat eats the rat.
Here, the AI learns that “cat” is followed by “eats” and then “the.” After “the,” there’s an equal chance of seeing “cat” or “rat.” By asking the AI for the next word and doing this over and over, it can make whole sentences.
Using the AI, you might get the same sentence as the one it learned from:
The cat eats the rat.
Or, you might get something different, like:
The rat.
The cat eats the cat eats the cat eats the rat.
Every time the AI sees “the,” it has to choose between “rat” and “cat.”
Of course, you would usually use much more text to teach the AI, but even with lots of text, there can be problems. For example, if you used all of Wikipedia, the AI might make a sentence like:
Explaining his actions, and was admitted to psychiatric hospitals because of Davis’s strong language and culture.
This sentence has more words and looks fancier, but it doesn’t make much sense because the AI isn’t thinking about the bigger picture. It’s only using the word right before to guess the next word. If the AI looked at two, three, or even four words before, it could make better sentences. But then, it might just copy big parts of the text it learned from. This makes us wonder: how often do the same four words show up in a row on Wikipedia?
Simplifying Language for Computers: Turning Words into Numbers
One of the big challenges in teaching AI about language is helping it understand what words mean and how they are related. How can we make a computer understand the meaning of words like “the” and “a” or “king” and “queen”? One way is to turn words into numbers, something computers are good at understanding.
Imagine giving numbers to words based on different scales. For example:
On a scale of -1 (masculine) to 1 (feminine):
king: -1
queen: 1
table: 0
mustache: -0.9
On a scale of -1 (mean) to 1 (nice):
wolf: -0.8
princess: 0.9
table: 0.1
gift: 1
On a scale of -1 (noun) to 1 (verb):
king: -1
speak: 1
pretty: 0
With enough scales like this, we can start to understand what a word means. But finding the right scales and giving them the right numbers is hard. To help with this, we use the computer’s strength of finding patterns. We teach it that words that show up together have related meanings. By looking at lots of text, the computer can find the scales and numbers all by itself. For example, “cat” and “rat” are both animals, and “eats” is a verb that works for them. But in a math book, you wouldn’t see “cat” or “rat” because their meanings are too different from what the book is about.
The scales the computer finds can be hard to understand. Some might be simple, like masculine/feminine, but others might only make sense when combined or when they represent more than one idea at once.
This method, called “word embedding,” turns words into a list of numbers called a vector. It helps connect language and meaning for computers.
Discovering Meaning with Numbers: How Word Embedding Shows Connections
When we turn words into numbers, we can see interesting patterns and even do math with word meanings. This new idea lets us play with word meanings in a mathematical way. For example, if we add the numbers for “USA” and “currency,” we might get a number close to the one for “dollar.” Here are some more examples:
"USA" + "capital" ≈ "Washington"
"eat" + "noun" ≈ "meal"
We can also try subtraction:
"king" - "man" + "woman" ≈ "queen"
"Washington" - "USA" + "England" ≈ "London"
These number patterns give us a special way to look at how words and their meanings are connected. We can also use this method to find words that are closely related or mean the same thing, which helps us learn more about how language works.
By turning language into numbers, word embedding gives us a new way to explore the complex world of meaning and connections in the words we use every day.
Teaching AI to Understand Language: Finding Relationships with Numbers
By turning words into numbers, we can help AI learn more about how words relate to each other. To do this, the AI needs to understand more about the bigger picture. With numbers, we can use estimates to help. Instead of just learning that “cat” comes after “eats,” the AI can learn things like “after an article and a noun, there’s often a verb,” “animals often eat, drink, and run,” “rats are smaller than cats,” and “you can only eat smaller things than you.” All of these relationships are shown as numbers.
The AI needs a lot of text to learn these relationships. These relationships are written as equations, like y = a * x1 + b * x2 + c, but with more inputs (the x1 values) and more parameters (a, b, and c). Now, the AI doesn’t just look at the chances of one word following another. It uses an equation for each scale (like masculine/feminine). This means the AI has hundreds of billions, or even trillions, of parameters.
How well the AI can understand the bigger picture depends on its size:
- 20 words would let it make simple sentences with the right structure.
- 100 words would let it explain a simple idea in a small paragraph.
- With a thousand words, it could have a conversation without getting lost.
- The biggest models, with around 20,000 words, can read a whole article, a short story, or have a long conversation while thinking about the whole context before making the next word.
In the end, the AI’s ability to learn relationships and think about the bigger picture depends on how big it is: a bigger model can understand more connections and think about a wider context.
The Strengths and Weaknesses of GPT: Understanding Advanced AI Models
GPT, especially the newest version, GPT-4, is excellent at creating text that sounds like it was written by a human. It’s great at connecting ideas, defending them, adjusting to the context, roleplaying, and avoiding contradictions.
But GPT has some downsides too. It might make up information or use its “imagination” when it doesn’t have the data it needs. If you ask it to solve a math problem, it might give you a wrong or completely made-up answer. Since it was only trained with data up to September 2021, GPT might make things up when asked about current events. To fix this problem, Bing Chat and Google Bard connect to search engines (Bing or Google) to get the latest information.
To get the most out of GPT, it’s important to use it for tasks that can handle some mistakes (like writing a marketing email) or tasks that can be checked by a non-AI program or a person. By knowing and working with GPT’s strengths and weaknesses, we can use this advanced AI model for many practical uses.
Understanding AI Thinking: Can GPT Really Think?
At first, it seems like GPT can’t think. It’s just a complex math equation that predicts the next word in a sequence. But when we look closer at the human brain and its many connections, we see some similarities between our brain and GPT, even though there are differences:
- Complexity: The human brain has 1,000 times more connections than GPT-4, so it can handle more complex situations.
- Continuous Learning: The brain keeps learning, even during conversations, while GPT stops learning before it starts interacting.
- Language Limitations: GPT works only with words, but it might be able to control a robot if it had enough data and used its understanding of meaning.
- Limited Input: GPT only uses text and misses nonverbal cues that are important in human communication.
There are also differences in behavior:
- GPT has trouble using logical rules consistently, like a young child, and doesn’t do well with math.
- GPT doesn’t have emotions but can copy emotional states from human interactions. This makes us wonder if this behavior means anything.
- The idea of consciousness is still debated. Most definitions focus on human thinking. If a program like GPT seemed just like a human, would it be considered conscious? The Chinese Room argument says that a computer following a program doesn’t really understand language or have consciousness.
So, whether GPT can think is still up for debate. This question encourages us to keep exploring how AI thinks and learns.
How GPT Affects Society: A New World for Knowledge Workers
It’s hard to predict the future, but it’s clear that GPT will bring big changes. Knowledge workers in many fields, like marketing, engineering, recruitment, and social work, will see their industries change because of GPT.
GPT’s impact is similar to how assembly lines changed craftsmanship, computers changed accounting, or mass media changed politics. Not all jobs will disappear right away, but some departments may need fewer people if one or two people can use GPT to do the work.
Society will feel these changes. Some people may need to change careers, learn to use GPT in their jobs, or face unemployment. New jobs will come up, either working directly with GPT, like Prompt Engineers who talk to the AI, or indirectly by helping create new products and companies.
This new time brings both good and bad. People with technical skills and the drive to start something new will probably do well, but those who don’t want to change or can’t get the resources to adapt might be in trouble. We don’t know the long-term effects of GPT on society yet, but it’s clear that big changes are coming.
GPT and Society: Fear of an AI Apocalypse?
Dystopian sci-fi often shows AI causing destruction with situations like:
- The Terminator scenario: A war AI with military power gets a survival instinct. When humans try to stop it, the AI fights back.
- The Paperclip Optimizer: Here, an AI’s job is to make as many paperclips as it can. After using up Earth’s resources, it targets the next available carbon source—humans. Or, the AI gets rid of humans to make paperclips without being bothered.
We should remember that GPT can only create text right now. While text can be powerful, GPT can’t directly hurt anyone. But, GPT could lead to more advanced systems, like AI-controlled robots or military decision-making tools.
We need to be careful as we keep working on AI technology and step in if things get out of control or aren’t regulated. Luckily, many AI experts are looking for ways to lower these risks, helping us move toward a safer future with AI.
source: https://confusedbit.dev/posts/how_does_gpt_work/
{
"prompt": "How Does GPT and ChatGPT work?, deepleaps.com, 4k, 8k, ultra highres, raw photo in hdr, sharp focus, intricate texture",
"seed": 2862633,
"used_random_seed": false,
"negative_prompt": "worst quality, low quality, normal quality, child, painting, drawing, sketch, cartoon, anime, render, 3d, blurry, deformed, disfigured, morbid, mutated, bad anatomy, bad art",
"num_outputs": 1,
"num_inference_steps": 1500,
"guidance_scale": 7.5,
"width": 512,
"height": 512,
"vram_usage_level": "high",
"sampler_name": "euler",
"use_stable_diffusion_model": "neverendingDreamNED_bakedVae",
"use_vae_model": "vae-ft-mse-840000-ema-pruned",
"stream_progress_updates": true,
"stream_image_progress": false,
"show_only_filtered_image": true,
"block_nsfw": false,
"output_format": "jpeg",
"output_quality": 75,
"metadata_output_format": "json",
"original_prompt": "How Does GPT and ChatGPT work?, deepleaps.com, 4k, 8k, ultra highres, raw photo in hdr, sharp focus, intricate texture",
"active_tags": [],
"inactive_tags": [],
"use_upscale": "RealESRGAN_x4plus",
"upscale_amount": "4",
"use_lora_model": ""
}