Open-Source Chatbot Vicuna Outperforming LLaMA and Alpaca, Rivals GPT-4 and Bard in Quality and Affordability
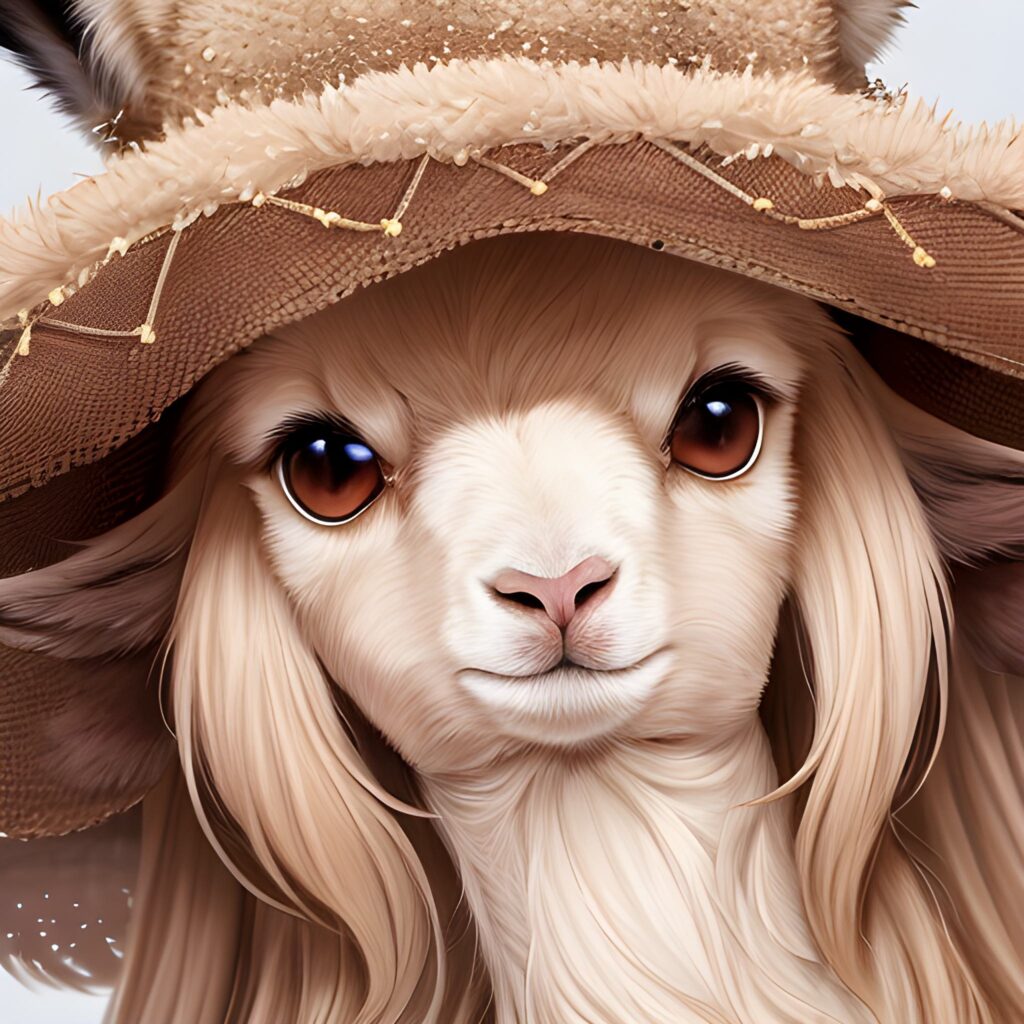
Vicuna outperforming LLaMA and Alpaca, Rivals GPT-4 and Bard, deepleaps.com, best quality, 4k, 8k, ultra highres, raw photo in hdr, sharp focus, intricate texture, skin imperfections, photograph of
A groundbreaking, open-source chatbot named Vicuna-13B has recently emerged as a strong competitor to OpenAI’s ChatGPT and Google’s Bard. Developed by a team of researchers from UC Berkeley, CMU, Stanford, and UC San Diego, this new chatbot shows promising performance, achieving over 90% quality of ChatGPT and outperforming other models like LLaMA and Stanford Alpaca in more than 90% of cases. Furthermore, the cost of training Vicuna-13B stands at a mere $300, making it an affordable alternative to the AI giants.
The Vicuna-13B chatbot is built upon the LLaMA framework, utilizing user-shared conversations collected from ShareGPT.com for fine-tuning purposes. According to the research team, Vicuna is capable of generating more detailed and well-structured answers compared to Alpaca, with a quality on par with ChatGPT after fine-tuning with 70,000 user-shared ChatGPT conversations.
Evaluating chatbot performance is no easy task, but the team has used GPT-4 as a preliminary judge for Vicuna-13B. Despite its initial success, the team acknowledges that the evaluation approach using GPT-4 is not yet rigorous and requires further research to build a comprehensive evaluation system.
Large language models (LLMs) have revolutionized chatbot systems, leading to unprecedented levels of intelligence like those seen in OpenAI’s ChatGPT. However, the lack of transparency in ChatGPT’s training and architecture has hampered research and innovation in this field. Vicuna-13B aims to address these concerns by offering an open-source, easy-to-use, and scalable infrastructure.
The team behind Vicuna-13B has made the training and serving code, as well as an online demo, publicly available for non-commercial use. They invite the AI community to interact with the demo to assess the chatbot’s capabilities and contribute to further improvements. With such a remarkable performance at a fraction of the cost, Vicuna-13B has the potential to democratize AI-powered chatbot technology, making it accessible to a wider audience.
How Vicuna-13B Delivers High-Quality AI Chatbot Performance at a Fraction of the Cost
Vicuna-13B, the open-source chatbot that boasts over 90% quality of ChatGPT and outperforms other models like LLaMA and Stanford Alpaca, has a unique training and serving process that maximizes efficiency and affordability. Developed by researchers from UC Berkeley, CMU, Stanford, and UC San Diego, the team behind Vicuna has shared insights into how they achieved such impressive performance at a fraction of the cost.
Training
The team began by collecting around 70,000 conversations from ShareGPT.com, a website where users can share their ChatGPT conversations. They then enhanced the training scripts provided by Alpaca to better handle multi-round conversations and long sequences. The training was done using PyTorch FSDP on 8 A100 GPUs in just one day.
To ensure data quality, they converted the HTML back to markdown and filtered out inappropriate or low-quality samples. They also divided lengthy conversations into smaller segments to fit the model’s maximum context length.
Memory optimizations, multi-round conversation handling, and cost reduction via spot instances were among the key improvements made to Stanford’s Alpaca model. By expanding the max context length from 512 to 2048, utilizing gradient checkpointing and flash attention, and employing SkyPilot managed spot to reduce costs, the team successfully trained the 13B model for around $300.
Serving
For serving the demo, the team implemented a lightweight distributed serving system capable of serving multiple models with distributed workers. This system supports flexible plug-in of GPU workers from both on-premise clusters and the cloud. By using a fault-tolerant controller and managed spot feature in SkyPilot, the serving system can work with cheaper spot instances from multiple clouds to reduce serving costs.
The team is currently working on integrating more of their latest research into the serving system. With such innovative training and serving solutions, Vicuna-13B has the potential to make AI chatbot technology more accessible, ushering in a new era of open-source AI development.
Evaluating Chatbots and Addressing Limitations: The Journey to Perfecting Vicuna-13B
Evaluating AI chatbots is a complex process, as it requires examining language understanding, reasoning, and context awareness. As AI chatbots become more advanced, current open benchmarks may no longer suffice. To address these challenges, the team behind Vicuna-13B, the open-source chatbot that rivals GPT-4 in quality and affordability, proposes an evaluation framework based on GPT-4 to automate chatbot performance assessment.
Evaluation Framework
The team devised eight question categories, such as Fermi problems, roleplay scenarios, and coding/math tasks, to test various aspects of a chatbot’s performance. GPT-4 generates diverse, challenging questions through careful prompt engineering, and the researchers then ask it to rate the quality of answers from five chatbots: LLaMA, Alpaca, ChatGPT, Bard, and Vicuna.
GPT-4 prefers Vicuna over state-of-the-art open-source models in more than 90% of the questions and achieves competitive performance against proprietary models. However, the team acknowledges that GPT-4 is not very good at judging coding/math tasks, and this evaluation framework is not yet a rigorous or mature approach.
Limitations
Similar to other large language models, Vicuna has limitations, such as struggling with reasoning or mathematics and accurately identifying itself or ensuring factual accuracy. It also has not been sufficiently optimized for safety, toxicity, or bias mitigation. To address safety concerns, the team uses the OpenAI moderation API to filter out inappropriate user inputs in their online demo.
Release
The team has released the training, serving, and evaluation code for Vicuna-13B on GitHub at https://github.com/lm-sys/FastChat. They have also released the model weights but do not plan to release the dataset.
Online Demo
Try their online demo here: https://chat.lmsys.org/
{
"prompt": "Vicuna outperforming LLaMA and Alpaca, Rivals GPT-4 and Bard, deepleaps.com, best quality, 4k, 8k, ultra highres, raw photo in hdr, sharp focus, intricate texture, skin imperfections, photograph of",
"seed": 5700684,
"used_random_seed": false,
"negative_prompt": "worst quality, low quality, normal quality, child, painting, drawing, sketch, cartoon, anime, render, 3d, blurry, deformed, disfigured, morbid, mutated, bad anatomy, bad art",
"num_outputs": 1,
"num_inference_steps": 1500,
"guidance_scale": 7.5,
"width": 512,
"height": 512,
"vram_usage_level": "high",
"sampler_name": "euler",
"use_stable_diffusion_model": "neverendingDreamNED_bakedVae",
"use_vae_model": "vae-ft-mse-840000-ema-pruned",
"stream_progress_updates": true,
"stream_image_progress": false,
"show_only_filtered_image": true,
"block_nsfw": false,
"output_format": "jpeg",
"output_quality": 75,
"metadata_output_format": "json",
"original_prompt": "Vicuna outperforming LLaMA and Alpaca, Rivals GPT-4 and Bard, deepleaps.com, best quality, 4k, 8k, ultra highres, raw photo in hdr, sharp focus, intricate texture, skin imperfections, photograph of",
"active_tags": [],
"inactive_tags": [],
"use_upscale": "RealESRGAN_x4plus",
"upscale_amount": "4",
"use_lora_model": ""
}