RedPajama 7B’s Instruct Model: A New Standard in HELM Benchmark Performance
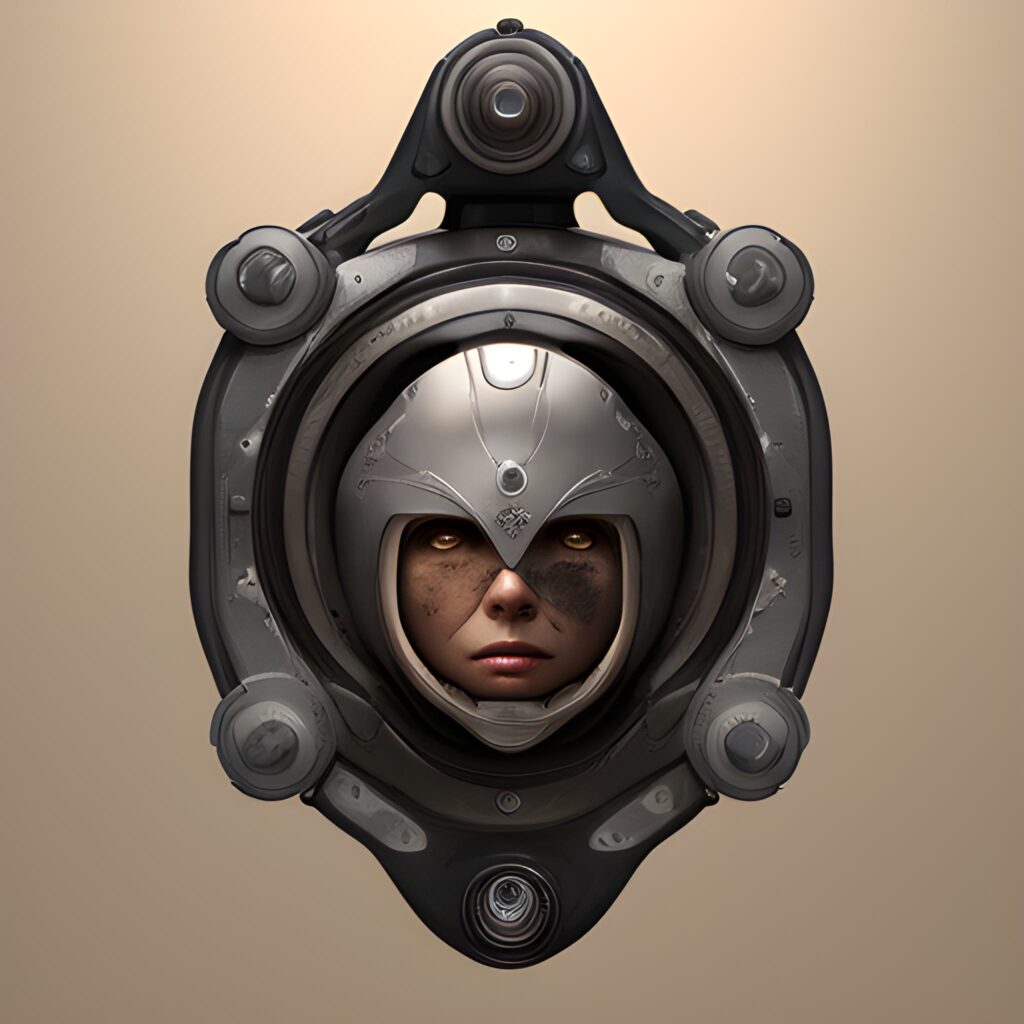
7B Instruct AI Model: A New Standard in HELM Benchmark Performance, deepleaps.com
In a recent development in the field of Artificial Intelligence (AI), the team behind the RedPajama project has released a novel collection of open-source models, reinforcing its commitment to fostering and understanding the conditions that enable high performance in AI.
The RedPajama project first came into the limelight in April when it introduced the RedPajama base dataset, a resource inspired by the findings of the LLaMA paper. This 5-terabyte dataset was swiftly adopted by the AI community, with thousands of downloads and applications in training over 100 models.
An outstanding collaboration ensued between RedPajama, the AAI CERC lab at Université de Montréal, EleutherAI, and LAION, involving the training of 3B and 7B models on the Summit supercomputer. This cooperative endeavour was part of the wider INCITE program award, entitled “Scalable Foundation Models for Transferable Generalist AI”.
The most recent milestone for the project has been the release of the RedPajama-INCITE family of models, which are equipped with instruct-tuned and chat versions, under the Apache 2.0 license.
The RedPajama-INCITE-7B-Instruct is a high-performing open model on HELM benchmarks, making it a suitable choice for a wide array of tasks. Its performance on HELM benchmarks surpasses that of the LLaMA-7B and other well-known open models such as Falcon-7B (Base and Instruct) and MPT-7B (Base and Instruct) by a margin of 2-9 points.
The RedPajama-INCITE-7B-Instruct is an instruction-tuned iteration of the base model. It excels at few-shot tasks and exhibits superior performance over leading open models of similar sizes, including Llama-7B, Falcon-7B (both base and instruct versions), and MPT-7B (both base and instruct versions) on HELM. It demonstrates robustness in performance on a variety of NLP tasks, sourced from both P3 (BigScience) and Natural Instruction (AI2). In order to maintain its integrity, the team went to lengths to decontaminate the instruct dataset against HELM in two comprehensive steps, removing tasks and instances that showed significant overlaps with any HELM validation example.
On the other hand, the RedPajama-INCITE-7B-Chat model, available in OpenChatKit, brings an innovative feature to the table: a training script for easily fine-tuning the model. Notably, this chat model is built exclusively on open-source data, maintaining a clean stance for use in open or commercial applications by avoiding distilled data from closed models such as those from OpenAI. Further efforts are being made to make this model available on a wide variety of hardware, including CPUs, mobile phones, and raspberry pi through the RedPajama.cpp and in collaboration with other projects like MLC LLM.
The third variant, RedPajama-INCITE-7B-Base, was trained on 1T tokens of the RedPajama-1T dataset. It releases with 10 checkpoints and open data generation scripts for complete reproducibility. This model, however, falls slightly short when compared to LLaMA-7B and Falcon-7B/MPT-7B on HELM by 4 and 1.3 points respectively. This shortfall was hypothesized to be due to FP16 training, the only precision available on Summit, prompting the team to plan on using higher precision in future training.
The project’s main objective is to offer more than just an impressive open model. Emphasis is also placed on ensuring complete reproducibility, with all details related to the creation of an open dataset, the recipe for building it, and the training process made readily available. The team recognizes that for AI to make significant strides, it is essential to involve a community of collaborators and developers. Thus, the team actively seeks feedback and suggestions from the broader AI community, to ensure a diverse and representative outlook is maintained.
As part of RedPajama’s future plans, they intend to balance the data mixture for each data slice better, and enlarge the portion of CommonCrawl in RedPajama2. The team will also look to include complementary slices from Pile v1 and Pile v2, and investigate further data deduplication strategies.
RedPajama is also looking to train larger models, particularly RedPajama-20B, and plans to launch a new training run using higher precision. These advancements aim to drive further progress, refine models and ultimately improve results in future iterations.
The progress made by the RedPajama project is indicative of the remarkable growth and innovation in the AI landscape. The project’s latest achievements promise not only advancements in the field but also a shared journey into the future of AI, built on collaboration and open-source principles.
https://www.together.xyz/blog/redpajama-7b
{
"prompt": "7B Instruct AI Model: A New Standard in HELM Benchmark Performance, deepleaps.com, Fantasy, Realistic, Photo, Surrealist, Unreal Engine",
"seed": 2793617161,
"used_random_seed": true,
"negative_prompt": "",
"num_outputs": 1,
"num_inference_steps": 25,
"guidance_scale": 7.5,
"width": 512,
"height": 512,
"vram_usage_level": "balanced",
"sampler_name": "euler",
"use_stable_diffusion_model": "Dreamshaper_3.32_baked_vae_clip_fix",
"clip_skip": false,
"tiling": "none",
"use_vae_model": "vae-ft-mse-840000-ema-pruned",
"stream_progress_updates": true,
"stream_image_progress": false,
"show_only_filtered_image": true,
"block_nsfw": false,
"output_format": "jpeg",
"output_quality": 75,
"output_lossless": false,
"metadata_output_format": "json",
"original_prompt": "7B Instruct AI Model: A New Standard in HELM Benchmark Performance, deepleaps.com",
"active_tags": [
"Fantasy",
"Realistic",
"Photo",
"Surrealist",
"Unreal Engine"
],
"inactive_tags": [],
"use_upscale": "RealESRGAN_x4plus",
"upscale_amount": "4"
}