Stability AI’s DeepFloyd IF: The New Generation Text-to-Image Model
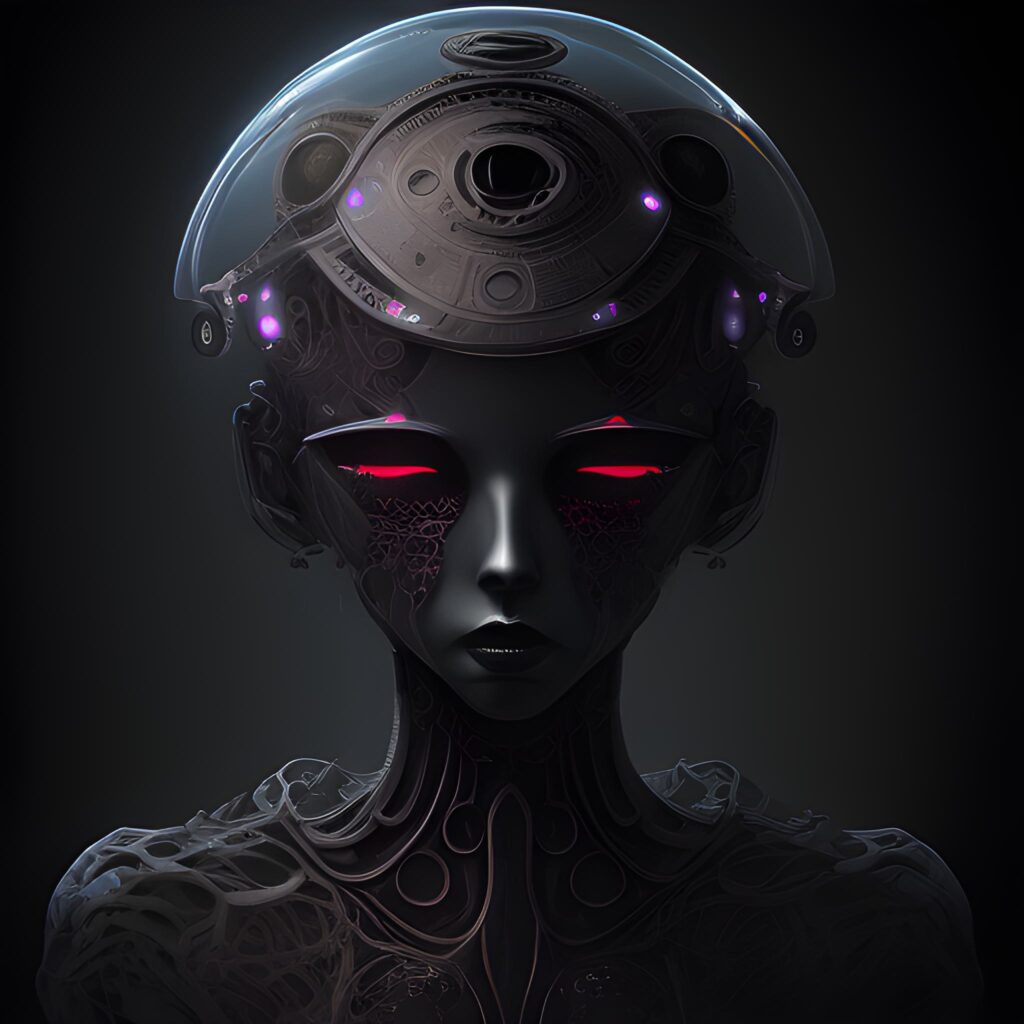
Stability AI, a renowned artificial intelligence research lab, has made yet another significant breakthrough in the field of AI. The company, along with its DeepFloyd multimodal AI research lab, has announced the release of DeepFloyd IF, a powerful text-to-image cascaded pixel diffusion model. The model is designed to revolutionize the way we integrate text into images and has the potential to transform various industries, including advertising, gaming, and film.
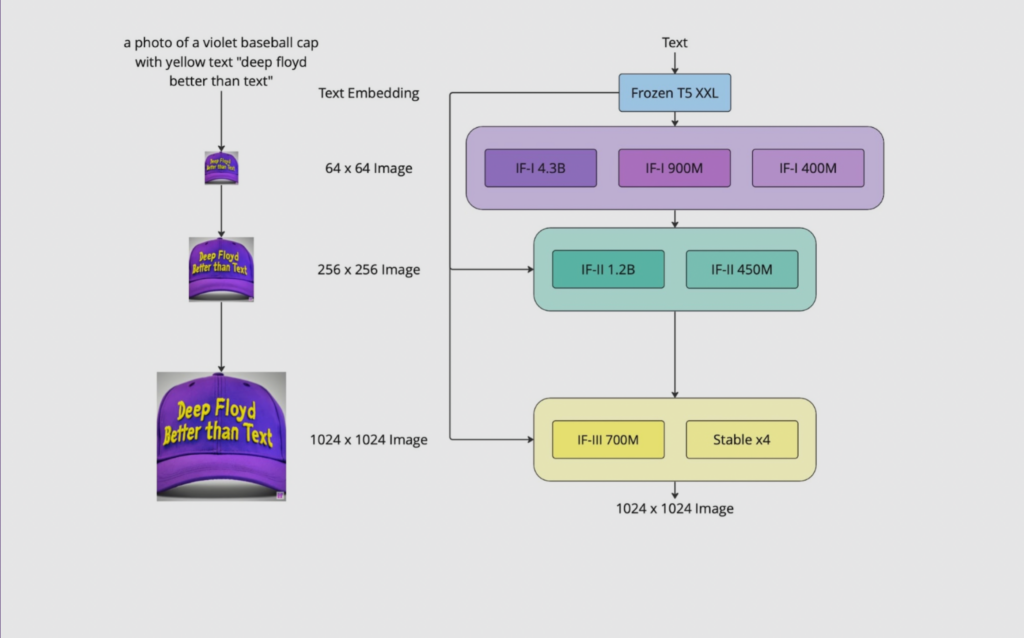
DeepFloyd IF is the latest text-to-image model to be released on a non-commercial, research-permissible license. This provides an opportunity for research labs to examine and experiment with advanced text-to-image generation approaches. Like other Stability AI models, the company intends to release a DeepFloyd IF model fully open-source at a future date.
The generation pipeline utilizes the large language model T5-XXL-1.1 as a text encoder. The pipeline also incorporates a significant amount of text-image cross-attention layers to provide better prompt and image alliance. With the intelligence of the T5 model, DeepFloyd IF generates coherent and clear text alongside objects of different properties appearing in various spatial relations. Until now, these use cases have been challenging for most text-to-image models.
DeepFloyd IF also possesses a high degree of photorealism. This property is reflected in the impressive zero-shot FID score of 6.66 on the COCO dataset (FID is a main metric used to evaluate the performance of text-to-image models; the lower the score, the better).
Another exciting feature of DeepFloyd IF is its ability to generate images with a non-standard aspect ratio, vertical or horizontal, as well as the standard square aspect. This capability will enable designers and artists to create images that are tailored to their specific needs.
The model also allows zero-shot image-to-image translations. Image modification is conducted by (1) resizing the original image to 64 pixels, (2) adding noise through forward diffusion, and (3) using backward diffusion with a new prompt to denoise the image. In inpainting mode, the process happens in the local zone of the image. The style can be changed further through super-resolution modules via a prompt text description. This approach gives the opportunity to modify style, patterns, and details in output while maintaining the basic form of the source image – all without the need for fine-tuning.
DeepFloyd IF is a modular, cascaded, pixel diffusion model. This means that the model consists of several neural modules whose interactions in one architecture create synergy. DeepFloyd IF models high-resolution data in a cascading manner, using a series of individually trained models at different resolutions. The process starts with a base model that generates unique low-resolution samples, followed by successive super-resolution models to produce high-resolution images.
DeepFloyd IF’s base and super-resolution models are diffusion models, where a Markov chain of steps is used to inject random noise into data before the process is reversed to generate new data samples from the noise. The diffusion is implemented on a pixel level, unlike latent diffusion models (like Stable Diffusion), where latent representations are used.
The model works in three stages: A text prompt is passed through the frozen T5-XXL language model to convert it into a qualitative text representation. In stage one, a base diffusion model transforms the qualitative text into a 64×64 image. This process is as magical as witnessing a vinyl record’s grooves turn into music. The DeepFloyd team has trained three versions of the base model, each with different parameters: IF-I 400M, IF-I 900M, and IF-I 4.3B.
To amplify the image, two text-conditional generative adversarial networks (TC-GANs) are used, which have been trained on a dataset of more than 50,000 high-quality images. These networks have the ability to generate images based on the given text description, and they were fine-tuned on the specific domain of fashion images.
The first TC-GAN, called the “text-to-image” network, takes in the text description and generates a low-resolution image, which is then passed to the second TC-GAN, called the “image refinement” network. The image refinement network enhances the low-resolution image and generates the final high-resolution image, which is then presented to the user.
The text-to-image network uses a technique called attention-based conditioning to generate images that accurately reflect the given text description. This technique allows the network to focus on specific parts of the text that are most relevant to generating the image. For example, if the text description mentions a red dress, the network will pay more attention to the words “red” and “dress” and generate an image that accurately reflects those attributes.
The image refinement network uses a technique called progressive growing to enhance the low-resolution image generated by the text-to-image network. This technique involves starting with a small image and gradually increasing the resolution of the image through multiple rounds of training. This allows the network to generate highly detailed images that are faithful to the original text description.
To ensure that the generated images are both realistic and diverse, the TC-GANs are trained on a diverse dataset of fashion images that cover a wide range of styles, colors, and patterns. The training dataset also includes images of people of different ages, genders, and ethnicities, which ensures that the generated images are inclusive and representative.
The TC-GANs are also equipped with a technique called style mixing, which allows the user to control the style of the generated image. This technique involves mixing the style of two different images from the training dataset to create a new style for the generated image. For example, if the user wants the generated image to have a vintage style, they can mix the style of a vintage image with the text description to create a new image with a vintage style.
Overall, the TC-GANs provide a powerful tool for fashion designers and retailers to generate high-quality images of clothing and accessories that accurately reflect the given text description. This can be particularly useful for e-commerce websites, where high-quality images are essential for attracting customers and driving sales.
However, it is important to note that the TC-GANs are not perfect and there are limitations to their abilities. For example, they may struggle to accurately generate images for text descriptions that contain complex or abstract concepts. Additionally, the generated images may not always be 100% accurate to the original text description and may require some manual editing.
Despite these limitations, the use of TC-GANs represents a significant step forward in the field of computer vision and has the potential to revolutionize the way that images are generated and used in the fashion industry and beyond.
Access to weights can be obtained by accepting the license on the model’s cards at Deep Floyd’s Hugging Face space: https://huggingface.co/DeepFloyd.
If you want to know more, check the model’s website: https://deepfloyd.ai/deepfloyd-if.
The model card and code are available here: https://github.com/deep-floyd/IF.
Everyone is welcome to try the Gradio demo: https://huggingface.co/spaces/DeepFloyd/IF.
Join us in public discussions: https://linktr.ee/deepfloyd
https://stability.ai/blog/deepfloyd-if-text-to-image-model
{
"prompt": "Stability AI's DeepFloyd IF: The New Generation Text-to-Image Model, deepleaps.com, Fantasy, Digital Art, Realistic, Surrealist",
"seed": 2071790772,
"used_random_seed": true,
"negative_prompt": "",
"num_outputs": 1,
"num_inference_steps": 25,
"guidance_scale": 7.5,
"width": 512,
"height": 512,
"vram_usage_level": "balanced",
"sampler_name": "euler",
"use_stable_diffusion_model": "faetastic_",
"use_vae_model": "vae-ft-mse-840000-ema-pruned",
"stream_progress_updates": true,
"stream_image_progress": false,
"show_only_filtered_image": true,
"block_nsfw": false,
"output_format": "jpeg",
"output_quality": 75,
"output_lossless": false,
"metadata_output_format": "json",
"original_prompt": "Stability AI's DeepFloyd IF: The New Generation Text-to-Image Model, deepleaps.com",
"active_tags": [
"Fantasy",
"Digital Art",
"Realistic",
"Surrealist"
],
"inactive_tags": [],
"use_upscale": "RealESRGAN_x4plus",
"upscale_amount": "4"
}