Meta Unveils ImageBind: A Groundbreaking Open-Source AI Model with Multimodal Abilities
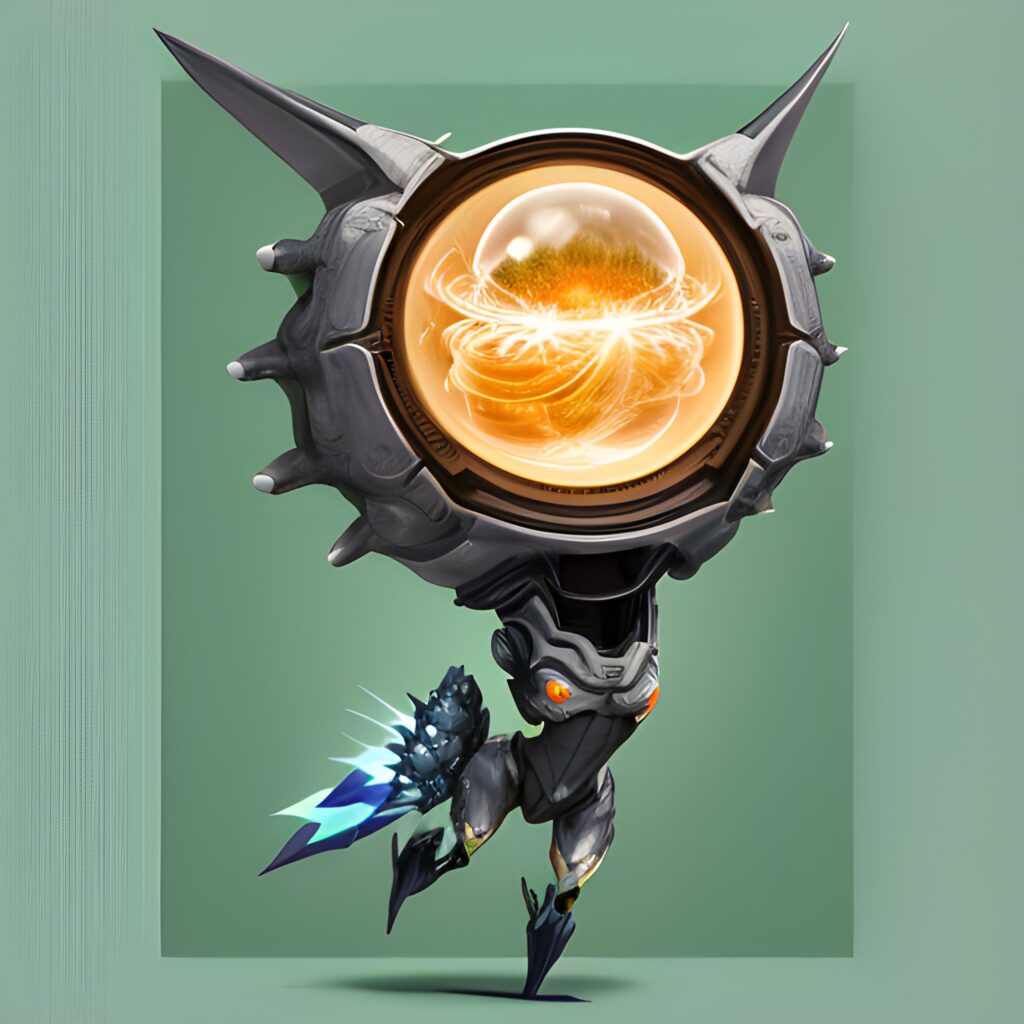
Meta unveils ImageBind, a groundbreaking artificial intelligence (AI) model capable of learning holistically and simultaneously from six different forms of information. This new approach pushes machines one step closer to humans’ natural ability to learn from various sensory inputs without the need for explicit supervision. ImageBind’s innovative technique enables machines to better analyze information and understand connections between objects, sounds, shapes, and other sensory data.
Pioneering Multimodal AI Systems
ImageBind is a multimodal AI model that forms part of Meta’s ongoing efforts to develop systems capable of learning from all types of data. As the number of modalities increases, researchers are presented with opportunities to develop new holistic systems, such as combining 3D and inertial measurement unit (IMU) sensors to create immersive virtual worlds. ImageBind’s potential applications also include memory exploration, content moderation, and creative design enhancement.
The model outperforms prior specialist models, learning a single embedding space across multiple modalities without training on every possible combination of data. This approach is crucial, as it would be infeasible for researchers to create datasets with all possible combinations, such as audio and thermal data from a bustling city or depth data and textual descriptions of seaside cliffs.
ImageBind’s potential extends beyond text, image, video, and audio, as it also encompasses depth, thermal, and IMU modalities. By building on the powerful visual features of other AI tools like DINOv2, ImageBind aims to further improve its capabilities in the future.
A Unique Learning Approach
Humans have the remarkable ability to learn new concepts from just a few examples. In contrast, traditional AI systems require extensive paired data for each modality. Ideally, a single joint embedding space could enable a model to learn visual features alongside other modalities.
ImageBind overcomes this challenge by leveraging large-scale vision-language models and extending their zero-shot capabilities to new modalities using naturally paired self-supervised data. The model uses images as a binding mechanism, allowing them to serve as a bridge between different modalities, such as text and motion. This unique approach enables ImageBind to align any modality that co-occurs with images, resulting in a more holistic interpretation of content.
Outperforming Previous Models
ImageBind’s image-aligned, self-supervised learning allows it to improve performance using very few training examples. The model exhibits new emergent capabilities or scaling behaviors that did not exist in smaller models but appear in larger versions. These include recognizing which audio fits a specific image or predicting a scene’s depth from a photo.
ImageBind’s scaling behavior improves with the strength of the image encoder, suggesting that larger vision models benefit non-vision tasks, such as audio classification. The model has also achieved new state-of-the-art performance on emergent zero-shot recognition tasks across modalities, even outperforming recent models trained specifically for those modalities.
The Future of Multimodal Learning
ImageBind offers exciting possibilities for creators by enabling the use of multiple modalities for input queries and retrieving outputs across other modalities. This capability could enhance image segmentation and object identification based on audio or even create animations from static images combined with audio prompts.
While the current research explores six modalities, introducing additional modalities, such as touch, speech, smell, and brain fMRI signals, may lead to richer, human-centric AI models. As the AI research community continues to explore multimodal learning, ImageBind serves as a crucial step in rigorously evaluating these models and revealing novel applications in image generation and retrieval.
Meta hopes that the research community will explore ImageBind and the accompanying published paper to discover new ways to evaluate vision models and develop innovative applications. With its holistic approach to learning, ImageBind marks a significant advancement in the field of AI and machine learning. By bridging the gap between human learning and machine understanding, ImageBind has the potential to revolutionize various industries and applications.
Innovative Applications Across Industries
ImageBind’s ability to analyze and understand connections across multiple modalities can have a significant impact on industries such as healthcare, entertainment, and education. For instance, healthcare professionals could leverage ImageBind to analyze patient data from various sources, such as medical imaging, audio recordings, and text, to make more informed decisions and provide personalized care. In the entertainment industry, ImageBind could be used to create immersive and interactive experiences by combining visual, audio, and other sensory inputs. Educators could use ImageBind to develop adaptive learning systems that cater to diverse learning styles, incorporating text, images, video, and audio elements.
Collaborative Development and Open-Source Access
Meta’s decision to open-source ImageBind encourages collaboration and experimentation within the AI research community. By providing access to this groundbreaking model, Meta aims to foster innovation and facilitate the discovery of new applications and techniques in multimodal learning.
As AI systems become increasingly sophisticated and capable of processing vast amounts of data from diverse sources, it is crucial to ensure that they are developed responsibly and ethically. Meta’s open-source approach to ImageBind promotes transparency and invites scrutiny from the broader research community, ensuring that the development of this transformative technology remains accountable and aligned with societal values.
Challenges and Future Directions
Despite its promising potential, ImageBind’s development also presents several challenges that must be addressed. One such challenge is the need for large-scale, diverse, and unbiased datasets to train and refine the model. Ensuring that ImageBind is trained on representative data is essential to avoid perpetuating biases and stereotypes that may be present in the data sources.
Additionally, the AI research community must continue to explore the ethical implications of multimodal learning and its potential impact on privacy and security. As AI systems like ImageBind become more capable of understanding and processing various forms of information, it is essential to implement safeguards that protect users’ rights and maintain trust in these technologies.
ImageBind represents a significant leap forward in the development of AI and machine learning systems. Its holistic approach to learning and ability to process multiple modalities brings machines closer to human-like understanding and opens the door to countless new applications and possibilities. By continuing to explore the potential of multimodal learning and addressing the challenges that arise, the AI research community can unlock the full potential of this transformative technology and shape a future where AI systems enhance human capabilities and improve our quality of life.
https://ai.facebook.com/blog/imagebind-six-modalities-binding-ai/
{
"seed": 65368189,
"used_random_seed": true,
"negative_prompt": "",
"num_outputs": 1,
"num_inference_steps": 125,
"guidance_scale": 7.5,
"width": 512,
"height": 512,
"vram_usage_level": "balanced",
"sampler_name": "euler",
"use_stable_diffusion_model": "revAnimated_v11",
"use_vae_model": "vae-ft-mse-840000-ema-pruned",
"stream_progress_updates": true,
"stream_image_progress": false,
"show_only_filtered_image": true,
"block_nsfw": false,
"output_format": "jpeg",
"output_quality": 75,
"output_lossless": false,
"metadata_output_format": "json",
"original_prompt": "Meta Unveils ImageBind: A Groundbreaking Open-Source AI Model with Multimodal Abilities, deepleaps.com",
"active_tags": [
"Fantasy",
"Realistic",
"Photo",
"Surrealist",
"Excited",
"Surprised"
],
"inactive_tags": [],
"use_upscale": "RealESRGAN_x4plus",
"upscale_amount": "4",
"prompt": "Meta Unveils ImageBind: A Groundbreaking Open-Source AI Model with Multimodal Abilities, deepleaps.com, Fantasy, Realistic, Photo, Surrealist, Excited, Surprised",
"use_cpu": false
}